Understanding Normally Distributed Data in Finance
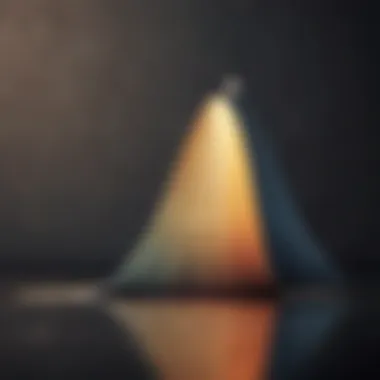
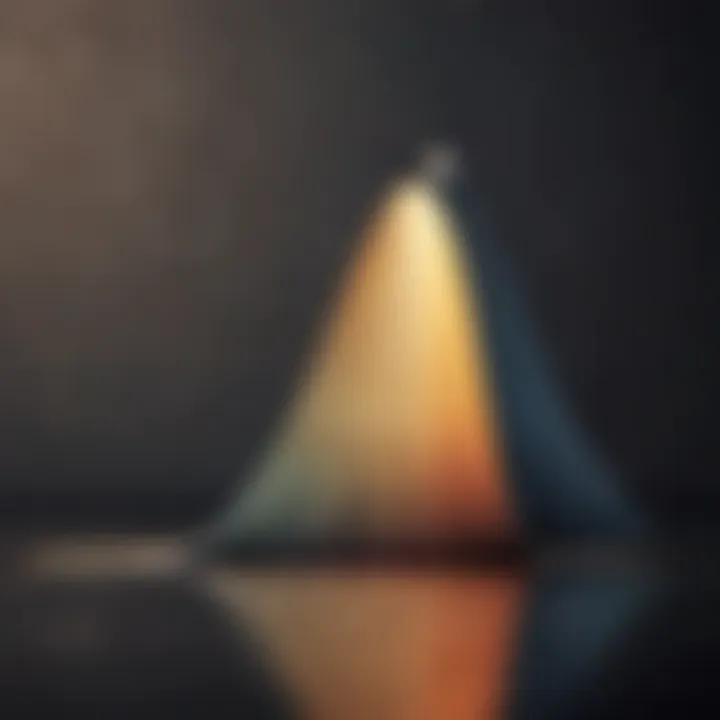
Intro
In the realm of finance, data is akin to the air we breathe—essential, yet often taken for granted. For investors and analysts, navigating this data effectively can make the difference between a well-informed decision and a costly mistake. One fundamental concept that emerges in the landscape of financial analytics is the idea of normally distributed data. Understanding this distribution is not just for academics; it is a practical toolkit for savvy investors looking to decipher the complex world of market movements.
Normal distribution is the bedrock of many statistical analyses. When we say a data set is normally distributed, we mean that it forms a bell-shaped curve when plotted graphically, with most of the data points clustering around the mean value. This shape suggests predictability, and predictability can be incredibly valuable in the often unpredictable world of finance.
The relevance of normal distribution in financial contexts stretches across various domains. Whether considering investment strategies or assessing risk profiles, recognizing how data behaves—how it is centered around the mean and varies—can lead to more robust decisions. Concepts like mean, median, and standard deviation come into play here, offering insights into performance metrics or market volatility.
As we explore these concepts further, it's crucial not to overlook the outliers and skewed data that can disrupt the narrative. These anomalies often carry significant implications, revealing risks or opportunities that a surface analysis might miss.
Understanding the Journey Ahead
This article aims to shed light on these intricacies. We will break down complex terms into digestible pieces, making it easier for readers to grasp critical insights. Practical examples and visual aids will enhance our discussion, ensuring clarity and engagement for everyone from investors to financial analysts.
"Statistics are like bikinis. What they reveal is suggestive, but what they conceal is vital." – Aaron Levenstein.
Throughout this exploration, we will address key financial jargon, tackle investment strategies informed by normally distributed data, and delve into risk assessment methods. In doing so, we hope to equip our audience with a comprehensive understanding that they can apply practically, thus translating theoretical knowledge into real-world success.
Foreword to Normal Distribution
In the realm of financial analytics, understanding normally distributed data sets is not just a luxury; it is a necessity. This section serves as the gateway to grasping how normal distribution underpins many analytical models used in finance. By laying this groundwork, readers will be better equipped to comprehend advanced concepts explored later in the article.
Normal distribution, characterized by its bell-shaped curve, becomes particularly relevant in finance where data typically cluster around a mean. The beauty of this statistical phenomenon lies in its ability to depict real-world scenarios, such as stock prices and returns, which often follow a normal distribution pattern.
Defining Normal Distribution
At its core, normal distribution refers to a probability distribution that is symmetric about the mean. This means that data near the mean are more frequent in occurrence than data far from the mean. You might say it showcases a kind of 'natural order' where most occurrences gather around an average value, while fewer instances lie at the extremes. Think of it like this: if you were to plot the heights of a large group of people, most would cluster around the average height with significantly fewer people being exceptionally tall or short.
In mathematical terms, normal distribution is defined by two parameters: the mean (average) and the standard deviation (a measure of spread). The mathematical representation often makes use of the notation ‘N(μ, σ^2)’ where μ represents the mean and σ^2 represents the variance. The resulting graph is perfectly symmetrical, where both sides mirror each other around the mean.
Importance in Financial Analytics
Normal distribution holds immense importance in the world of finance for several compelling reasons:
- Risk Management: Many financial models, particularly those related to risk assessment, are built on the assumption that returns follow a normal distribution. This simplifies the evaluation of risk and potential returns, which can significantly aid in decision making.
- Predictive Modelling: By assuming normality in returns, analysts can make predictions based on historical data. For example, they may use historical average returns and standard deviations to forecast future volatility and performance.
- Performance Metrics: Various performance metrics like Sharpe Ratio, which compares an investment’s excess return to its standard deviation, assume a normally distributed return profile.
Understanding normal distribution shapes the lens through which investors and analysts assess risk and make informed decisions. A misjudgment or lack of comprehension regarding this distribution can cause major hiccups in financial forecasting.
"The greatest error is not to have enough, but to have too much confidence in your estimates based on normality."
As the article progresses, we'll uncover not only the beauty of normal distribution but also its limitations and areas of application within the world of finance.
Mathematical Properties of Normal Distribution
Understanding the mathematical properties of normal distribution is crucial for anyone involved in financial analytics. This section sheds light on the underlying elements that define the normal distribution, enabling financial professionals to make informed decisions based on quantitative metrics.
Characteristics of the Normal Curve
The normal curve, often depicted in the shape of a bell, holds several notable characteristics that facilitate its application in finance. Here are a few key features:
- Symmetry: The curve is symmetric about the mean, which indicates that data points are equally likely to occur above or below it. This property helps analysts infer probabilities and makes assumptions easier to manage.
- Concentration of Data: Most values cluster around the mean, tapering off symmetrically towards the extremes. This means that most financial returns or metrics often lie close to the average, which minimizes the chances of significant anomalies interfering with trend analysis.
- Asymptotic Behavior: The tails of the curve approach the horizontal axis but never actually touch it. This characteristic highlights that extreme events, though rare, still have a non-zero probability of occurring, emphasizing the importance of considering outliers in financial assessments.
Mean, Median, and Mode
When it comes to a normally distributed dataset, the mean, median, and mode all reside at the same central point. This property is what gives the normal distribution its beloved title of being "unimodal."
- Mean: Often referred to as the average, the mean is vital for purposes like calculating expected returns. It provides a clear measure of central tendency, ensuring investors gauge the general performance of their assets.
- Median: Since the median represents the midpoint of the dataset, its value can sometimes offer a better reflection of where things stand when distributions are skewed. In finance, using both median and mean can safeguard against the misinterpretation of skewed data, especially in income distribution.
- Mode: Although it may seem less relevant in financial modeling, the mode can help identify the most frequent return or observed value in datasets. Identifying the mode can add depth to analysis, especially in real estate or fixed-income securities where typical values carry significant weight.
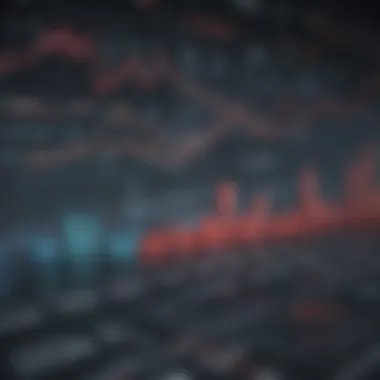
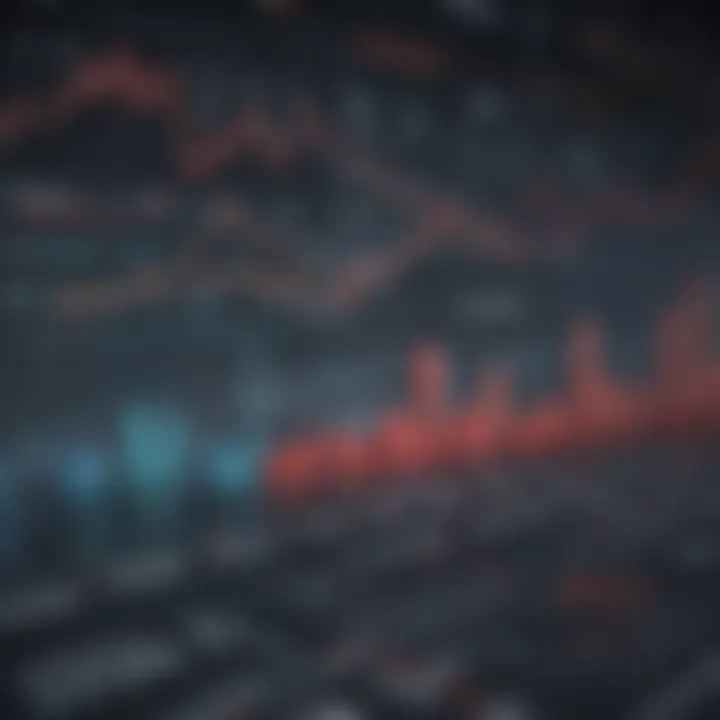
Standard Deviation Explained
The standard deviation is a principal aspect of understanding any normal distribution. In finance, it serves as a key measurement of risk, pointing to the variability or volatility of returns around the mean. Here's why it's important:
- Measure of Variation: A low standard deviation means that data points tend to be close to the mean, while a high one indicates that they are spread out over a larger range of values. This insight is crucial for investors looking to assess the risk profile of various investment options.
- Confidence Intervals: In practice, when you combine the mean and the standard deviation, you can create a confidence interval. This interval is instrumental in investment decision-making, giving stakeholders an idea of where they might expect returns to fall within a set probability range.
- Risk Assessment: By understanding the standard deviation, analysts can evaluate potential risks associated with individual assets or entire portfolios. For example, a portfolio with high variability might deter risk-averse investors but could also indicate potential for high rewards.
In financial analytics, grasping these mathematical properties of normal distribution is fundamental, acting as a linchpin for sound investment strategies and risk management practices.
In summary, familiarity with the mathematical properties of normal distribution not only fosters a robust understanding of data patterns but also equips financial professionals to navigate complex market dynamics with greater confidence.
Applications in Finance
The application of normally distributed data in finance is profound and multifaceted. The principal reason this distribution is favored in financial analytics is its capacity to simplify the complexity of real-world data. Investors and analysts frequently grapple with large data sets, and a standard bell curve helps in interpreting risks and identifying opportunities in a more digestible way.
Investment Strategy Modeling
Portfolio Optimization
Portfolio optimization is one of the key aspects of ensuring that investments yield the maximum returns relative to the risk taken. By using normally distributed data, analysts can estimate returns on a portfolio of investments. A characteristic of this optimization is the efficient frontier, where optimal portfolios with the highest expected return for a given level of risk can be identified.
This method becomes beneficial as it allows for a systematic choice between various asset classes, helping investors to align their portfolios more closely with their investment objectives and risk tolerance. The understanding that returns on investments tend to follow a normal distribution enables sophisticated modeling techniques that can project potential future returns.
However, while portfolio optimization through this lens is beneficial, it has its pitfalls. Significant shifts in market conditions, or unforeseen events, can lead to assumptions based on historical data being misleading. For instance, in 2008, many models that employed normal distribution did not account for the financial crisis, leading to significant undervaluation of risks.
Asset Allocation
Asset allocation is another critical aspect where normally distributed data plays a role. It involves distributing investments among various financial categories, like stocks, bonds, and cash. The underlying principle is that different asset categories do not move in tandem; when stocks may be down, bonds might be up, balancing the risk across the whole portfolio.
The key characteristic of asset allocation is the emphasis on diversification, aimed at maximizing returns while minimizing risks. Investors often use historical return distributions to guide their allocation decisions, making it a valuable strategy in this article. Based on the data, one can analyze how a mixed portfolio might behave under various economic conditions, an essential exercise in preempting risk.
Yet, while asset allocation strategies can be robust, they are influenced by the normality assumption of returns. Distributions that exhibit tails heavier than the normal distribution can result in potential losses that models based on normality can underestimate. Hence, recognizing how non-normal data affects asset allocation is significant for astute decision-making.
Risk Assessment Techniques
Value at Risk (VaR)
Value at Risk (VaR) is a standard metric employed in risk management to estimate the potential loss in value of a portfolio over a defined period for a given confidence interval. It hinges on the assumption that returns are normally distributed, which means it can be calculated more straightforwardly without extensive simulation models.
The key characteristic of VaR is that it provides a clear picture of potential financial loss, allowing firms to measure and manage risk effectively. This model is advantageous because it offers a concise metric that can be communicated easily among stakeholders, facilitating better risk management practices.
Nonetheless, the reliance on the normal distribution can lead to underestimating risks associated with extreme market fluctuations—what they call “tail risk.” This limitation has spurred a range of alternative measures and adjustments to the basic VaR model to account for real-world irregularities.
Stress Testing
Stress testing is an invaluable tool used to evaluate how a financial institution might fare under extreme economic scenarios. It helps in analyzing the resilience of a portfolio against potential shocks or drastic changes in market conditions. This technique often involves assessing the potential outcomes based on historical data and modeling hypothetical adverse conditions.
A notable characteristic of stress testing is its focus on extreme market conditions, which are often disregarded in models based purely on normal distribution. Due to these characteristics, it has garnered significant attention in the realm of financial regulation and risk management.
Yet, as effective as stress tests can be, they are only as good as the scenarios presented. If the scenarios don’t comprehensively adapt to possible variations in economic strains, the results may provide a false sense of security about financial stability.
Behavioral Analysis of Investors
Behavioral analysis involves studying how psychological influences and cognitive biases affect how investors behave in the market. Understanding these behaviors can offer valuable insights into market movements that deviate from what might be expected based purely on statistical normality. This aspect is particularly important in determining why market prices can oscillate more dramatically than what normally distributed models predict.
By looking at the factors that influence decisions—like fear, greed, and herd mentality—investors can better navigate the unpredictability of the market. These insights can lead to better strategies that consider both statistical data and human behavior, making for astute decision-making in uncertain environments.
"Investors need to balance the rational with the emotional. Understanding human behavior can lead to more informed decisions in the realm of finance."
The intersection of normally distributed data and behavioral trends creates a textured landscape of financial analytics, offering investors a more robust way to navigate their investment strategies, risk assessments, and overall market participation.
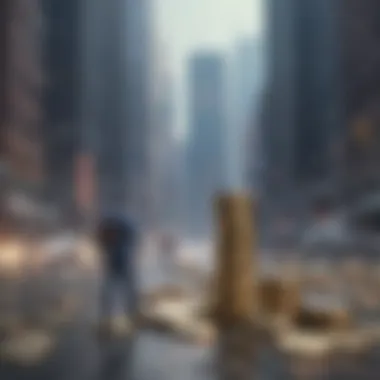
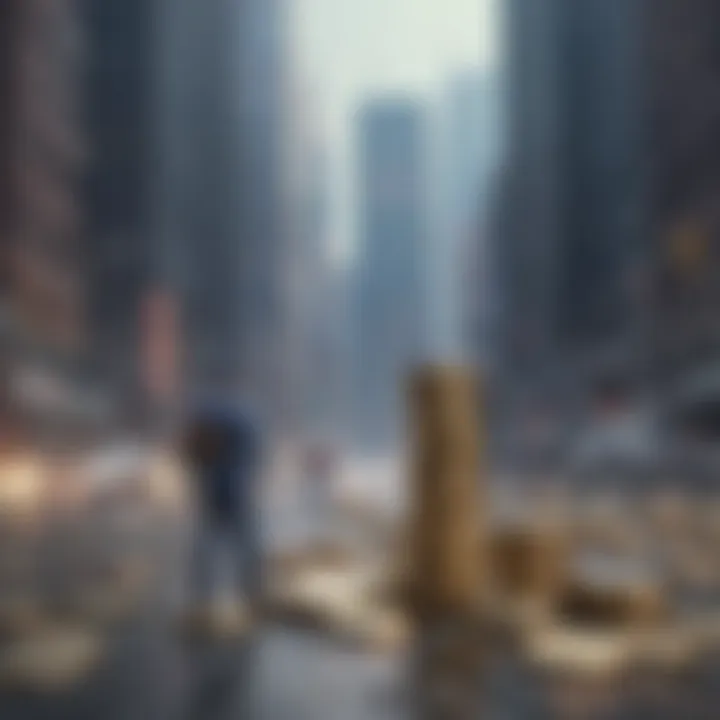
Interpreting the Normal Distribution in Financial Data
Understanding how to interpret the normal distribution in financial data is critical for a variety of reasons. For investors, financial advisers, and analysts alike, the normal distribution provides a framework for making informed decisions based on historical data trends. The characteristics of a normal distribution, such as symmetry around the mean and the predictable behavior of values within certain standard deviations, help in assessing the risk and potential returns associated with different financial instruments.
Financial markets are inherently unpredictable, yet the normal distribution offers a lens through which to view risk and performance. Recognizing when data deviates from this standard can signal critical changes in market conditions or individual asset performance and can guide timely decision-making.
Identifying Skewed Data
When working with financial datasets, it’s essential to be able to identify skewness; this refers to a situation where data points lean to one side rather than being symmetrically distributed. In a perfect normal distribution, the data is evenly distributed along the mean. However, it’s not uncommon in financial settings to confront skewed data which can lead to misinformed risk assessments.
For instance, in stocks, if we find that the returns display a right skew, it might indicate that there are a few instances of extremely high returns that pull the mean away from the median. This skewness could tempt an investor to perceive higher performance than actually exists, leading to exaggerated expectations. Here are some key indicators for spotting skewed data:
- Histogram Shapes: Using histograms can visually reveal whether your data follows normal distribution or if it's leaning left or right.
- Statistical Tests: Tests like the Shapiro-Wilk or Jarque-Bera can statistically determine the presence of skewness.
- Analysis of Quartiles: A comparison of the 75th percentile to the 25th percentile can indicate skewness.
By identifying skewed data, financial analysts can take necessary actions, like adjusting their models or portfolios to mitigate potential losses.
Impact of Outliers
Outliers are another critical aspect to examine when interpreting normal distribution in financial data. An outlier is an observation that is significantly different from the rest of the data points. These could arise from measurement error, data entry error, or true variability in the underlying data.
In many financial datasets, a single outlier can substantially impact the mean and standard deviation, leading to misleading insights. For example, if a hedge fund faces a freak market event causing a significant loss or gain, that single data point can swing averages unrealistically. Here are considerations when dealing with outliers:
- Assess the Cause: Determine whether the outlier is valid data or a byproduct of error.
- Rethink the Model: Sometimes, using median instead of mean can offer a better measure of central tendency, especially when outliers are present.
- Robust Statistical Methods: Consider using statistical techniques that minimize the influence of outliers, ensuring a more accurate interpretation of data.
Understanding both skewness and outliers doesn't just refine analysis; it enables more educated approaches to investment strategy and risk management. Recognizing these elements can significantly enhance an investor's capacity to navigate the unpredictable waters of financial markets. If you want to dive deeper into skewness and outliers, resources like en.wikipedia.org provide valuable insights.
Limitations of Normal Distribution in Finance
Understanding the limitations of normal distribution is crucial for financial analysts, investors, and advisors. While normal distribution provides a foundational framework for analyzing data, its application in finance can often lead to misinterpretations. Without a doubt, the financial world has a fair number of surprises that a smooth bell curve simply can’t catch.
For one, normal distribution assumes that data points are symmetrically distributed around the mean. However, in finance, markets are not inherently predictable. Events such as financial crises or market crashes are often extreme and cannot be accounted for when relying solely on normal distributions. Therefore, relying exclusively on this statistical model can be both misleading and dangerous.
Failing to Capture Extreme Events
Extreme events, often referred to as black swan events, are outliers that lie well outside the anticipated outcomes predicted by normal distribution. These are the market tremors and tremors that are most vividly remembered, like the 2008 financial crisis or the dot-com bubble burst at the turn of the millennium. These occurrences create what’s termed as fat tails in statistical models, meaning that the probability of extreme variations is significantly greater than what a normal distribution would suggest.
Think of it like this: if someone argued that a financial instrument is safest simply because its historical price movements fit a normal distribution, they might be in for a rude awakening. Market shocks or plunge—those instances when a stock drops 50% in a matter of days—happen much more frequently than the bell curve would imply. In short, normal distribution doesn't predict these sudden downturns or surges well enough.
"Investing is often more about psychology than statistics. The market doesn't always play by the rules that statistics set."
Misleading Assumptions
Normal distribution in finance tends to create a set of assumptions that investors and analysts often take at face value. One common assumption is that returns are independent and identically distributed (i.i.d). However, financial returns can exhibit patterns and correlations that violate this assumption. Correlation can change, especially during crises or periods of market volatility.
Moreover, it is also presumed that the data set will start with a known mean and standard deviation—the very basis upon which predictions are made. This isn’t always the case in financial analytics. Market sentiments, investor behaviour, and various external variables continually affect these parameters, contradicting the normal distribution's static approach.
These misleading assumptions can mess with risk evaluations, portfolio predictions, and strategy formulation. When decisions are based on an overly simplified model like normal distribution, they can yield erroneous conclusions. Investors who do not critically assess their data are courting trouble, wandering deeper into a quagmire of financial missteps.
Thus, while normal distribution offers helpful insights, it is essential to remember its boundaries. Investors must couple this understanding with robust alternative analyses to better navigate the complexities of financial markets.
Visualizing Normal Distribution
Visualizing normal distribution plays a crucial role in financial analytics. It provides a clear representation of data characteristics, revealing patterns, trends, and potential anomalies within data sets. By translating complex statistical concepts into graphical formats, investors and financial analysts can easily grasp essential insights that would otherwise be buried in numbers. Moreover, an effective visualization not only enhances comprehension but also aids in decision-making processes, allowing for a more informed approach to investments and risk assessments.
A well-constructed visualization can emphasize important statistical parameters such as the mean, median, and standard deviation. These measurements serve as critical indicators of the distribution's behavior, offering insights into the expected returns and risks associated with various securities and investment strategies. Recognizing the shape and spread of the normal curve can help analysts anticipate market moves and adjust their strategies accordingly.
Using Graphs and Charts
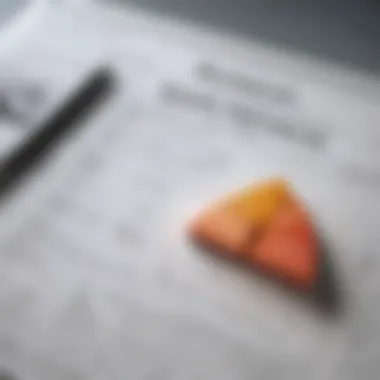
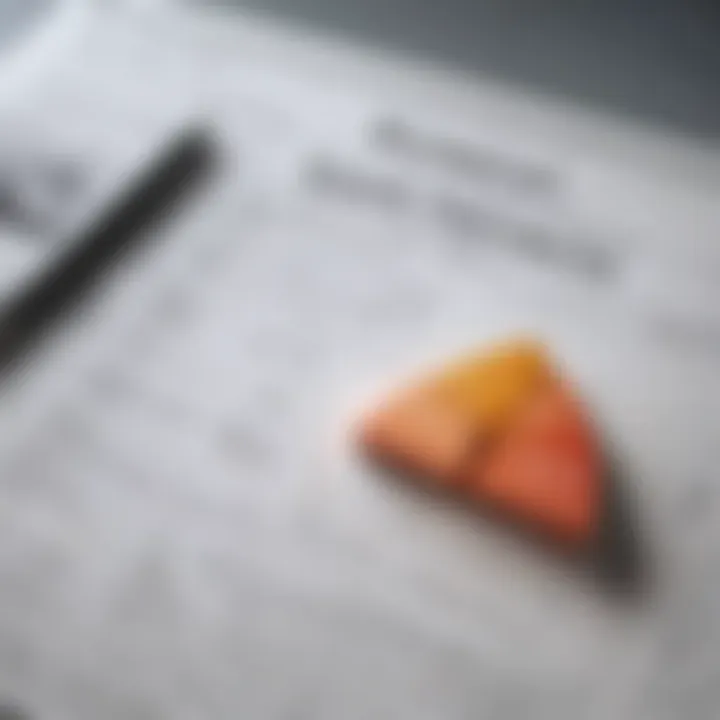
Graphs and charts are the bread and butter of data visualization. They encapsulate large amounts of information in a digestible format. The bell curve, familiar to many, is the hallmark of normal distribution and elegantly illustrates how data is dispersed around the mean. Key points worth noting include:
- Highlighting the Mean: The highest point of the curve indicates the mean, showing the most likely value in a dataset.
- Standard Deviation: Extending from the mean, the curve's width illustrates the standard deviation. A wider curve signals greater variability, while a narrower one suggests uniformity in the data.
- Confidence Intervals: Shading sections of the graph can represent confidence intervals, signifying where the majority of data points are likely to fall.
Using software tools like Excel, Tableau, or Python libraries (like Matplotlib and Seaborn), one can easily generate these graphs. As a visual learner, seeing the numbers laid out graphically can often clear the fog that statistics may create. It opens doors to pattern recognition and data trend spotting.
Illustrative Case Studies
A few well-documented case studies can unfold the real-world implications of visualizing normal distribution.
- Stock Market Returns: Analysts often plot the historical returns of a specific stock to assess its normality. By doing so, they can deduce patterns, volatility, and the probability of certain return thresholds being met. For instance, using a line graph to visualize monthly returns over five years can illuminate whether returns tend to hover around the mean or sporadically dip and rise due to market forces.
- Real Estate Prices: Visualization of real estate pricing can greatly aid estate dealers in understanding price dynamics. When plotting data of property prices within a region, the distribution can show whether it's a buyer's or seller's market. A normal distribution suggests a balanced market, whereas a skew might indicate bubbles or depressions.
Using these case studies, professionals can derive actionable insights that guide investment decisions.
"The clarity that comes from visualizing data in financial contexts is invaluable—it’s not just about numbers, but about understanding the stories they tell."
In summary, visualizing normal distribution serves as an essential tool in financial analytics, enhancing understanding and fostering better decision-making. By employing various graphical methods and analyzing case studies, investors and financial analysts can navigate the complexities of financial data more effectively.
Case Studies of Normal Distribution in Financial Markets
Understanding how normally distributed data operates in various segments of the financial markets is pivotal for investors and analysts alike. These case studies not only illustrate practical applications of theoretical concepts but also bring to life the implications of normal distribution in real-world scenarios. Knowing how data behaves—whether it conforms to a normal distribution or strays from it—can dramatically influence investment strategies and risk assessments.
Stock Market Analysis
When analysts evaluate stock prices, they often utilize normal distribution to make predictions about price movements. The assumption here is that stock returns should roughly cluster around the mean, usually reflecting a rational market where news and events are absorbed systematically. Through the lens of normal distribution, an investor can calculate expected returns and their associated risks.
In a recent study of a large tech company's stock over a decade, it was found that daily returns followed a pattern closely resembling a normal distribution. Here's how:
- Mean Returns: On average, the stock returned about 8% annually.
- Standard Deviation: The annual volatility was observed to be around 15%, indicating how much returns strayed from the mean.
This allows for effective risk management since investors can utilize the standard deviation to assess the probability of extreme events. For instance, many investors assume that returns should stay within two standard deviations of the mean about 95% of the time. If the stock price moves beyond this, it raises a flag for potential review.
Moreover, considering factors like overall market conditions, a market downturn can cause significant shifts in return distributions. During periods of high volatility, such as the 2008 financial crisis, the assumption of normality can break down—suggesting that reliance solely on past data can mislead investors.
Real Estate Valuation
Real estate markets can also demonstrate normal distribution characteristics. However, certain variables like location, property type, and economic conditions can skew the dataset. For example, if you analyze several residential properties within a confined area, their values could display a bell-shaped curve if they exhibit similar features and appeal to comparable buyers.
Let's think through a case study involving suburban homes:
- Average Home Value: Say the average value is estimated at $300,000.
- Standard Deviation: If the average variance from this mean is about $30,000, it indicates most properties fall between $240,000 and $360,000.
- Application: This helps investors identify under- or over-valued properties.
However, factors that influence prices—like unique architectural features or sudden neighborhood development—can introduce skewness into the data. When valuing a property, one must be aware of this potential deviation; real estate assessments that assume normal distribution may overlook significant factors like buyer sentiment or economic shifts.
"In the realm of finance, a clear grasp of how normal distribution frames asset pricing can set the creed apart from mere participation."
Ending and Future Research Directions
In wrapping up our discussion on normally distributed data sets in financial analytics, it's critical to recognize the movement of data trends in tandem with evolving financial landscapes. The principles of normal distribution form a bedrock for numerous analytical practices, guiding investors in their decision-making processes and risk assessments. A proper understanding of normal distribution can significantly shift how professionals forecast future events and interpret historical data.
Summarizing Key Insights
To distill the essence of our exploration:
- Normal distribution is foundational in shaping financial models and instruments. Understanding its characteristics allows both novice and seasoned investors to approach analysis with a sharper lens.
- The mean, median, and standard deviation are crucial metrics that help analysts gauge risk and returns in investment portfolios.
- Applications spread across various domains, including stock market analysis and real estate valuation, demonstrating the versatility and relevance of normally distributed data sets.
- Acknowledging limitations, such as the inability to capture extremes, encourages a more cautious approach in interpretation. Understanding this provides a refinement in strategies deployed across markets.
Exploring Beyond Normality
While the discussion around normal distribution demonstrates its utility, finance isn’t always about standard patterns. Recognizing patterns outside of the norm can lead to groundbreaking insights. Situations that deviate from normal distribution —like unexpected market crashes or high volatility—challenge conventional wisdom. It’s here that looking ‘beyond normality’ opens avenues for innovative analytical strategies.
In summary, as we look to the unpredictable nature of financial markets, the relevance of understanding normally distributed datasets is only heightened. Future research should pivot towards enhancing tools that can model risk beyond established norms.
As the field continues to evolve, investors and analysts are encouraged to embrace a more comprehensive approach that considers both the predictability of normal distribution and the irregularities posed by outlier events.