Exploring Predictive Analytics: Types and Applications
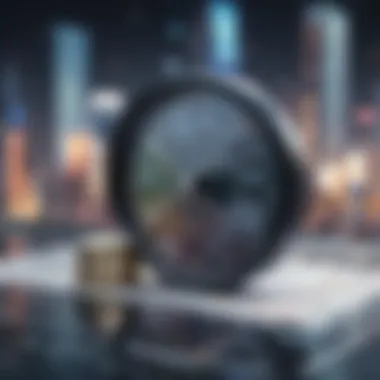
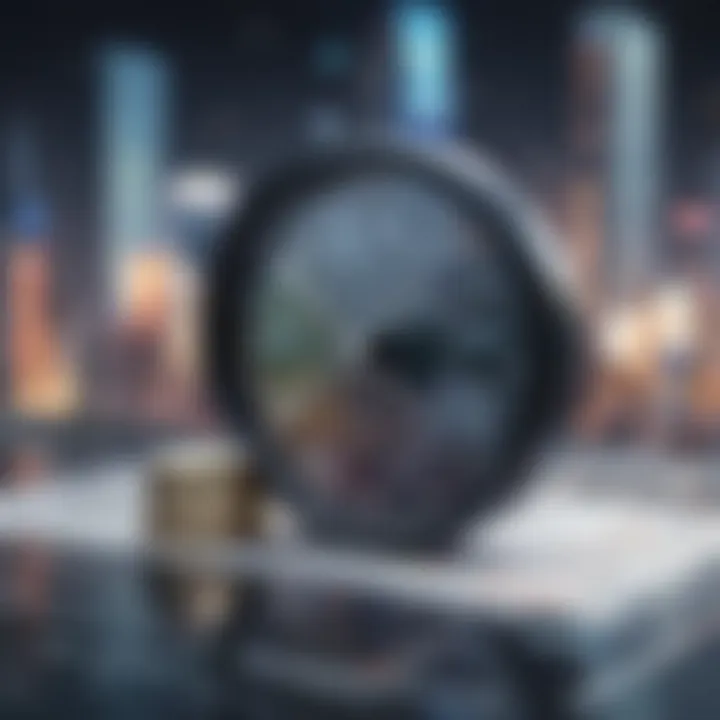
Intro
Navigating the vast arena of predictive analytics requires not just an understanding of its methods and applications, but also a solid grasp of the language that permeates this field. Whether you’re an investor keen on leveraging these techniques for financial gain, a financial advisor aiming to assist your clients, or an entrepreneur poised to seize market opportunities, having a well-defined lexicon is crucial.
Predictive analytics encompasses a variety of techniques and methodologies that forecast future outcomes based on historical data. Before we dissect the methodologies and applications further, it is important to lay a foundational understanding of key financial terms and investment jargon commonly encountered in this field.
Investment Dictionaries
Understanding the terms and phrases unique to predictive analytics is vital for clear communication and informed decision-making. This section will guide you through key financial terms and provide insights into the often bewildering jargon of investing.
Key Financial Terms
In the world of predictive analytics and finance, certain terms are ubiquitous. Familiarity with these terms can significantly aid in understanding better ways to utilize analytics. A few key terms include:
- Predictive Modeling: The process of using data mining and machine learning techniques to build a model that predicts future outcomes based on historical data.
- Regression Analysis: A statistical method for estimating the relationships among variables, commonly used in predictive analytic models to forecast trends.
- Time Series Analysis: Involves statistical methods to analyze time-ordered data points; crucial for understanding financial metrics over time.
- Big Data: Refers to the immense volume of data that can be analyzed to reveal patterns, trends, and associations, particularly in predicting outcomes.
A good grasp of these terms sets the stage for deeper insights into how predictive analytics works.
Understanding Investment Jargon
Investing often requires specialized vocabulary. Knowing these terms helps prevent miscommunication and misunderstandings. Here are some phrases you might encounter:
- Risk Appetite: An investor's willingness to take risks in pursuit of returns. Understanding risk appetite is essential for effective investment strategies.
- Asset Allocation: The strategy of dividing investment portfolios among different asset categories, such as stocks, bonds, and cash. It can heavily influence the results of predictive modeling.
- Market Volatility: Refers to the rate at which the price of assets fluctuates. Understanding its patterns is essential for making predictions.
Equipping yourself with this jargon allows for a more nuanced engagement in discussions surrounding predictive analytics.
"In the financial world, clarity in language often translates to clarity in strategy."
As we delve deeper into the types of predictive analytics, the methodologies behind them, and their diverse applications, having a relevant vocabulary in your toolkit will enhance not just your comprehension but also your ability to apply this knowledge effectively.
Preface to Predictive Analytics
In an age defined by information overload, the ability to sift through large volumes of data and extract actionable insights has become paramount. This is where predictive analytics steps in, offering a nuanced approach to understanding future trends based on historical data. Throughout this article, we will delve into the multiple types of predictive analytics, giving investors and analysts the tools they need to navigate an increasingly complex landscape.
Engaging with the nuances of predictive analytics is no longer optional for savvy investors or business leaders—it’s essential. The very fabric of decision-making processes within various industries is woven tightly with the threads of prediction and analysis.
Definition and Overview
Predictive analytics, at its core, involves using statistical algorithms and machine learning techniques to identify the likelihood of future outcomes based on historical data. Simply put, it’s about making educated guesses rooted in data patterns. This approach can be illustrated as reading the leaves to understand the shifting winds rather than relying solely on intuition or gut feelings.
Predictive analytics is not just about programming algorithms or crunching numbers. It’s about creating a story from data. By examining past events and trends, businesses can better prepare for what’s to come. For instance, retail companies often analyze purchase histories to forecast demand for various products in upcoming seasons, which allows them to optimize inventory levels accordingly.
Importance in Today's Data-Driven World
The relevance of predictive analytics in today's data-driven world cannot be overstated. We live in an era where data is flowing like water, and the ability to harness that data to predict future outcomes gives businesses a competitive edge.
- Carving a Competitive Edge: Firms harnessing predictive analytics are not just reacting to market trends; they are anticipating them. This capability can significantly improve operational efficiency and strategic planning. For instance, organizations leveraging predictive models can optimize supply chains, ensuring resources are allocated efficiently.
- Enhancing Customer Relationships: Understanding customer behavior through predictive analytics allows businesses to tailor their marketing strategies effectively. Brands can segment their audiences based on predicted purchasing behaviors, enabling them to create personalized marketing campaigns that resonate better with potential customers.
- Risk Mitigation: In sectors such as finance and insurance, predictive analytics aids in identifying risks and preparing contingency plans before issues arise. For example, insurance companies can use predictive models to assess the risk levels associated with various clients, determining suitable premium rates while managing their exposure effectively.
"The future belongs to those who prepare for it today." - Malcolm X
Descriptive Analytics
Descriptive analytics plays a pivotal role in the realm of predictive analytics. It serves as the foundation upon which more complex analysis types can be built. By providing insights into what has happened in the past, descriptive analytics helps organizations make sense of their data and informs the strategic decisions they will take.
For investors and financial advisors, understanding descriptive analytics is not just an academic exercise; it is a crucial tool that aids in evaluating the performance of assets, understanding market trends, and ultimately making informed decisions. \nWhile some may view this type of analysis as merely outlining historical data, its importance cannot be overstated, especially when combined with diagnostic and predictive analytics to foster deeper insights.
Understanding Descriptive Analytics
Descriptive analytics encompasses the analysis of historical data to identify trends, patterns, and anomalies. It is a clarion call to all investors to know their past so they can navigate their future better. Unlike predictive analytics, which attempts to forecast future outcomes, descriptive analytics only focuses on summarizing what has already occurred. This process often involves data aggregation techniques that convert raw data into a comprehensible format, transforming numbers into meaningful narratives.
This type of analytics is prevalent across various industries. For instance, in finance, it's common to see performance metrics displayed in reports. In retail, businesses might analyze sales data to understand buying preferences. All these activities share a common ground: they describe outcomes that can help unravel the reasoning behind business performance.
Common Techniques and Tools
The approaches within descriptive analytics can be diverse. Here’s a closer look:
- Data Visualization: Using graphs, charts, and dashboards to illustrate data, making it accessible and easier to comprehend.
- Descriptive Statistics: These include measures like mean, median, mode, and standard deviation, which help summarize and describe relevant characteristics of a dataset.
- Reporting: Regularly generating reports on key performance indicators (KPIs) to keep stakeholders informed about the current state of the business.
- Data Mining: This involves sifting through large sets of data to identify patterns and correlations that might not be readily apparent.
"Descriptive analytics is not just about numbers; it is about the story those numbers tell."
Some popular tools utilized for descriptive analytics include Tableau and Microsoft Power BI, both known for their robust visualization capabilities. Excel remains a staple for many, providing functionality to analyze and summarize data easily.
In summary, descriptive analytics is indispensable for investors and business leaders alike. It not only charts the course for future predictive models but also embraces a deeper understanding of current operations by analyzing past performances. With this foundation laid, analysts can then delve into diagnostic analytics, uncovering the why's behind the data.
Diagnostic Analytics
Diagnostic analytics plays a pivotal role in deciphering the underlying reasons behind past outcomes. Unlike its descriptive counterpart, which merely outlines events, diagnostic analytics digs deeper to unearth anomalies, trends, and patterns that might otherwise go unnoticed. This approach empowers investors and analysts by providing insights that help pinpoint the causes of financial successes or pitfalls. In the increasingly competitive business arena, leveraging diagnostic analytics can be akin to having a compass in a foggy landscape, directing stakeholders toward smarter, data-driven decisions.
What is Diagnostic Analytics?
At its core, diagnostic analytics involves the examination of data to provide answers to why something happened. It employs various methods and tools to dissect data sets, highlighting the factors that lead to specific outcomes. For instance, if a company's sales dipped in a quarter, diagnostic analytics would look at sales data, marketing efforts, economic conditions, and competitor actions to determine the root causes of that decline.
This type of analytics relies on a multitude of techniques, such as data mining, correlation analysis, and even advanced statistical models. It goes a step further than just reporting, focusing on causal relationships rather than simple correlations. This can involve more than just quantitative analysis; qualitative data, like customer feedback and market surveys, can also provide context that enriches findings.
Applications of Diagnostic Analytics
The realm of diagnostic analytics is broad, extending its influence across various sectors. Here are a few illustrative examples:
- Finance: In finance, diagnostic analytics helps analysts understand fluctuations in stock prices by examining historical data trends, market indicators, and economic conditions. For example, a drop in the market can be linked to increased interest rates through careful data analysis.
- Healthcare: Hospitals can utilize diagnostic analytics to identify the causes of readmission rates. By analyzing patient data, they can detect patterns, such as specific treatments that consistently lead to complications.
- Retail: Retailers can analyze customer purchasing behavior to determine why specific products are underperforming. This could involve looking into customer complaints, sales trends, and promotional effectiveness.
"Diagnostic analytics is like a detective — it peels back layers to reveal hidden truths."
In practice, the insights gleaned from diagnostic analytics lead to actionable strategies. For instance, if a bank learns that younger customers are shifting to online banking but struggling with usability, they can then revise their website's user experience to better cater to this demographic.
Moreover, one crucial consideration is that while diagnostic analytics provides valuable insights, it must be carried out with a keen awareness of data limitations and bias. Misinterpretation can lead to misguided decisions, so a critical eye is necessary in analyzing the results. This interplay between data and discernment forms the backbone of effective decision-making in any organization.
Predictive Analytics
Predictive analytics has emerged as a vital tool in the modern data landscape, playing a significant role across various sectors. In essence, it enables organizations to forecast future outcomes based on historical data patterns and trends. For investors, understanding predictive analytics can lead to astute decisions that can minimize risks while maximizing returns.
The importance of predictive analytics in this environment cannot be overstated. With the continuous evolution of technology, it provides insights that are not just hypothetical but rooted in statistical rigor. Organizations that harness its capabilities can identify opportunities before they become apparent to competitors; this can be the difference between seizing a lucrative investment and lagging behind.
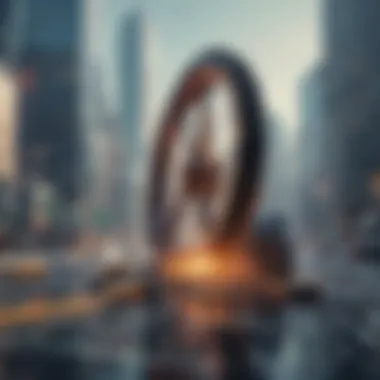
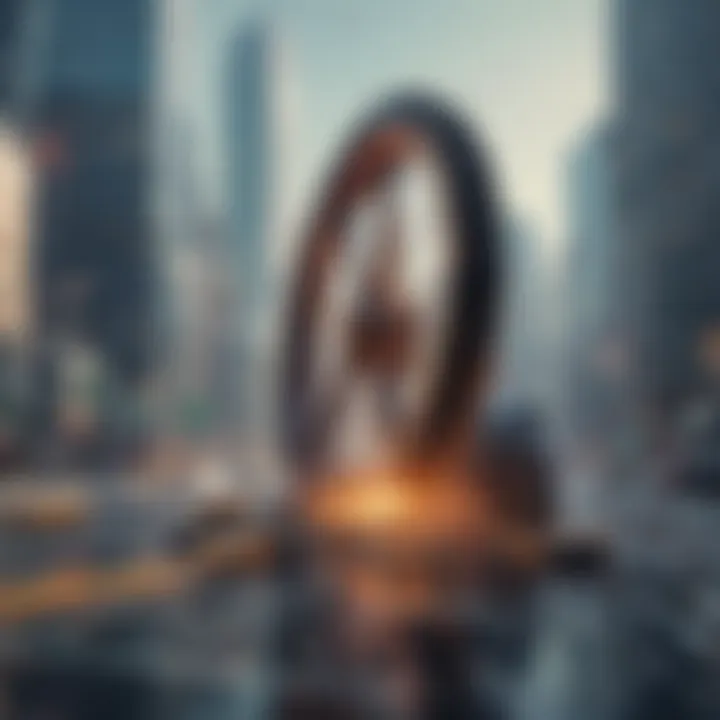
Moreover, predictive analytics is more than a crystal ball. It's about being proactive rather than reactive. Investors who adapt predictive analytics into their strategies can detect shifts in market dynamics and consumer behaviors, allowing them to pivot when necessary. This foresight fosters resilience, particularly in economic downturns when quick adaptations can safeguard investments.
Key Features of Predictive Analytics
Predictive analytics is characterized by several key features that contribute to its effectiveness:
- Data Mining: This forms the backbone of predictive analytics. By sifting through vast stores of data, businesses can uncover hidden patterns that may escape initial scrutiny.
- Statistical Algorithms: They are employed to analyze data. These algorithms help in identifying correlations and predicting future trends based on existing data.
- Machine Learning Models: These models learn from past data automatically, improving their predictive capabilities over time without additional programming.
- Data Visualization: Effective presentation of data is crucial. Tools that visualize predictive models help stakeholders grasp complex trends quickly.
"Predictive analytics helps in turning bewildering volumes of data into actionable insights, translating patterns into predictions that help guide decision-making processes."
These features work together to enhance an organization’s ability to predict outcomes accurately. By integrating diverse data sources and employing advanced techniques, predictive analytics empowers enterprises to make timely and informed choices.
Statistical Techniques Utilized
Within the realm of predictive analytics, a multitude of statistical techniques is utilized to yield insights that drive decision-making:
- Regression Analysis: Often employed to estimate relationships among variables, this technique helps in forecasting a dependent variable (like sales) based on one or more independent variables (like advertising spend).
- Time Series Analysis: This technique focuses on historical data to forecast future points in the series, beneficial for financial analysts projecting stock prices or sales revenues.
- Classification Techniques: These algorithms, like decision trees or logistic regression, categorize data into predefined classes, aiding in predicting outcomes like customer churn.
Incorporating these techniques enables organizations to create models that not only make predictions but also gauge their reliability and accuracy. As investors and analysts apply these methodologies, they can anticipate market trends, consumer behaviors, and other critical factors, thus sharpening their competitive edge.
Understanding the importance and features of predictive analytics sets a strong foundation for analyzing how it can be effectively implemented and utilized in various contexts. By doing so, investors can tread carefully through the tumultuous waters of today’s markets.
Prescriptive Analytics
In the realm of data analysis, prescriptive analytics emerges as a powerful tool that takes the practice of making predictions a step further. Unlike descriptive analytics or diagnostic analytics that explain past events or predict future occurrences, prescriptive analytics prescribes actions based on data insights. This type of analytics provides organizations with recommendations on possible actions they can take to achieve desired outcomes, thereby steering decision-making towards success.
Businesses today are inundated with data, but it's not merely the quantity that matters—it's how that data is processed and utilized. Understanding prescriptive analytics becomes crucial in environments where rapid responses are necessary, and the margin for error is slim. It serves as a guiding light, enabling organizations to optimize operations, improve resource allocation, and ultimately drive results.
Defining Prescriptive Analytics
Prescriptive analytics can be best understood as the intersection of data analysis and decision-making advice. At its core, it uses techniques from statistical modeling, machine learning, and operations research to analyze potential future scenarios and recommend a course of action.
To put it plainly, prescriptive analytics gives you not just the ‘what’—like predicting sales figures—but the ‘how’—advising on pricing strategies, inventory levels, or marketing approaches based on those predictions. It synthesizes a vast amount of data to provide actionable insights, making the decision-making process more informed and strategic.
Features of prescriptive analytics include:
- Simulation and Optimization: Enables organizations to simulate different scenarios and identify optimal strategies based on varied inputs.
- Algorithms and Models: Leverages advanced algorithms to generate recommendations tailored to specific situational contexts.
- Real-Time Processing: Processes vast datasets in real-time to keep recommendations relevant and timely.
Operationalizing Outcomes
Implementing prescriptive analytics in a business environment requires a thoughtful approach to ensure it delivers on its promise. The journey starts with clearly defined objectives. Companies must first identify what outcomes they are seeking to achieve through prescriptive analytics: is it increasing sales, improving customer retention, or optimizing logistics? Setting these goals forms a roadmap.
Once objectives are established, the next step is data accumulation and integration. High-quality and relevant data serves as the backbone of any prescriptive model. Various data sources, including customer interactions, transaction histories, and market trends, should be harnessed and consolidated. Handling this stage with care is critical, as the integrity of the recommendations is directly proportional to the quality of the input data.
After gathering the necessary data, businesses should employ robust analytics tools and technologies. Advanced analytics platforms equipped with machine learning capabilities are vital here, as they can process information and generate prescriptions efficiently. However, it’s not just about technology; it's equally important to have domain expertise to interpret the analytics results meaningfully.
In practice, organizations should consider the following strategies:
- Iterative Testing: Regularly test models against actual outcomes to fine-tune recommendations.
- Feedback Loops: Establish mechanisms for continuous feedback on the recommendations made, allowing for adjustments based on real-world results.
- Stakeholder Involvement: Engage all relevant stakeholders from the start—sales, marketing, finance, and technology—to ensure a holistic perspective on the output.
"Success in the operationalization of prescriptive analytics hinges on blending data insights with human intuition and expertise."
The application of prescriptive analytics can ultimately foster not only improved decision-making but also strategic foresight, helping organizations to navigate complexities and competition in their respective fields.
Causal Analytics
Causal analytics represents a critical component within the realm of predictive analytics. While other types often focus merely on correlations or trends, causal analytics digs deeper, aiming to uncover the underlying cause-and-effect relationships that influence outcomes. This is particularly of interest to investors, analysts, and decision-makers, as understanding these dynamics can significantly enhance strategic planning and risk assessment.
In today's complex, data-rich environment, the need for causal analysis has become paramount. Organizations seek precise reasons behind observable changes. Whether it’s an uptick in a product's sales or a sudden drop in stock price, grasping the specifics of causality enables stakeholders to devise proactive strategies. The importance of this type of analytics cannot be overstated; it equips users with the ability to not only understand what is happening but also why it is happening.
The Role of Causal Analytics
The role of causal analytics extends far beyond theoretical discussions. Practically, it influences decisions that can lead to tangible financial success or failure. By applying causal analytics, organizations can:
- Identify Root Causes: This type of analysis helps in pinpointing why an event occurs, rather than just observing its aftermath. For instance, if a company finds that increased marketing spend leads to higher sales, causal analytics will reveal how much of that increase can be attributed directly to the marketing efforts versus external factors like seasonality or competitor actions.
- Enhance Predictive Models: When integrated into predictive models, causal analytics refines these frameworks to provide more reliable forecasts. Decision-makers can utilize enriched data sets, filled with causal relationships, which enhances overall predictive accuracy.
- Support Decision-Making: Investors heavily rely on understanding causal links to make informed decisions regarding portfolios, asset allocations, and risk management.
Effective utilization of causal analytics involves a multi-step process, often starting with hypothesis formulation followed by rigorous testing, which can involve a variety of approaches, including experiments and observational studies.
Methodological Approaches
Several methodological approaches exist to effectively engage with causal analytics. Each presents unique strengths depending on the context in which they are applied. Here are some notable strategies:
- Randomized Controlled Trials (RCTs): Considered the gold standard, RCTs involve randomly assigning subjects into treatment and control groups. This minimizes biases, providing high-quality insights into cause and effect.
- Observational Studies: When RCTs are impractical, observational studies serve as an alternative. Analysts can observe existing data to identify potential causal relationships, using statistical techniques to control for confounding variables.
- Path Analysis: This method charts out directional pathways illustrating how different variables influence one another. It helps in visualizing complex relationships and understanding indirect effects.
- Structural Equation Modeling (SEM): This approach allows for testing complex relationships and causal pathways simultaneously, which can be beneficial in understanding multifaceted interactions within data sets.
- Time Series Analysis: By examining data points collected over time, this method can reveal trends and cycles, and inform on causation particularly in economic and financial models.
Causal analytics, when executed thoughtfully, can yield a wealth of insights, driving better-informed decisions. It turns raw data into a narrative, guiding investors and stakeholders through the labyrinth of data richness to actionable strategies that optimize financial or operational outcomes.
"Understanding the cause behind the effect is like having a map in a dense forest; it leads you through the thicket of uncertainty toward informed decision making."
In the fast-paced world of data-driven decision making, mastering causal analytics offers a vital edge to those willing to invest the effort into understanding and applying these methodologies.
Real-Time Analytics
In the landscape of predictive analytics, real-time analytics stands out as a critical element that offers organizations the ability to make swift, informed decisions based on data as it arrives. This immediacy isn't just a luxury; it’s a necessity in modern markets where old data can quickly become obsolete. By leveraging real-time insights, businesses can respond to customer behavior, operational challenges, and market shifts almost instantaneously. The convergence of data streams allows deeper visibility into operational efficiencies and customer engagement strategies, knitting businesses closer to their customer base while steering them clear of potential pitfalls.
Conceptual Framework of Real-Time Analytics
Real-time analytics can be understood as the practice of constantly analyzing data as it is generated. This approach is vital for companies navigating the chaos of today's fast-paced economy. Key components of the conceptual framework of real-time analytics include:
- Data Streams: Continuous flow of real-time data from various sources. This might include social media, transaction records, or sensor data from IoT devices.
- Processing Frameworks: Utilizing powerful algorithms and technologies to process incoming data on-the-fly. Technologies like Apache Kafka or Apache Storm often play a significant role here.
- Actionable Insights: The end goal of real-time analytics is transforming raw data into actionable insights. This can mean adjusting supply chain logistics in response to unexpected demand or modifying marketing strategies as customer preferences shift.
Moreover, businesses should consider the need for a solid data architecture, including both input mechanisms and data storage solutions that support velocity—the rate at which data is generated and analyzed.
Technological Requirements
To implement real-time analytics effectively, companies must invest in the right technological foundations. Here are key technological requirements:
- High-Performance Data Ingestion: Companies often utilize tools like Apache NiFi or Amazon Kinesis to ensure that data is captured as it comes in, without delays.
- Real-Time Processing Engine: The use of a dedicated processing engine such as Apache Flink, Spark Streaming, or even cloud-native services like Google Cloud Dataflow is essential to parse and process data immediately.
- Storage Solutions: A blend of traditional and NoSQL databases can be necessary, where systems can hold not just structured data, but also unstructured data, enabling a more flexible querying capability. Solutions such as MongoDB or Cassandra can be highly effective for this.
- Visualization Tools: Real-time dashboards that depict analytics visually are vital for stakeholders to interpret the data quickly. Examples include Power BI or Tableau that can process and visualize real-time data efficiently.
In light of these considerations, companies looking to embrace real-time analytics should approach it with a well-planned strategy and a clear understanding of the technologies involved.
"In today's world, data that isn't analyzed instantly loses its potency; the clock is ticking every second."
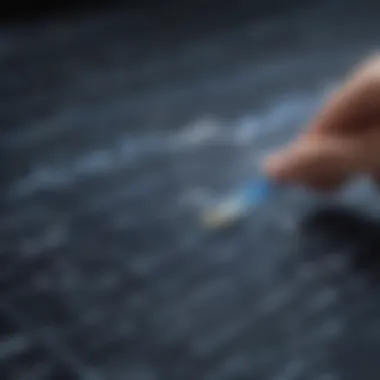
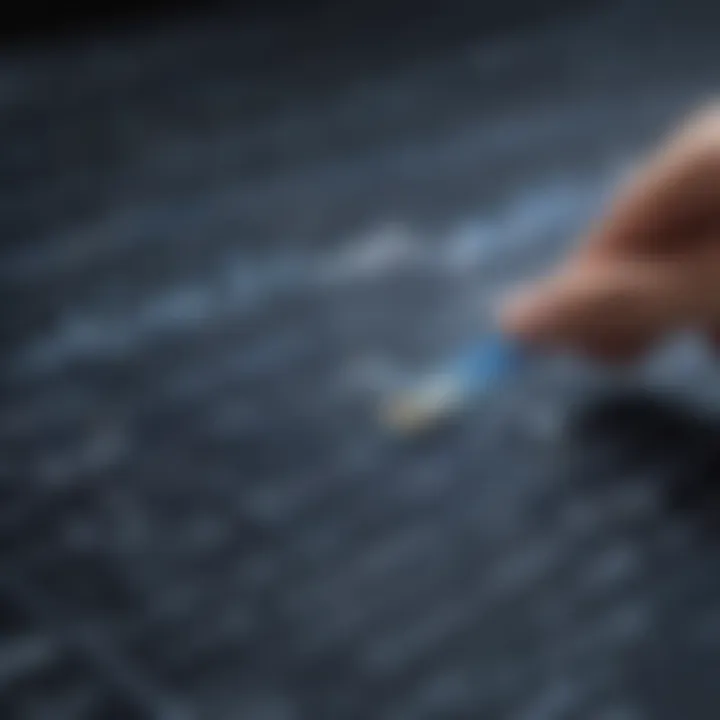
By harnessing the right tools and methodologies, organizations can unlock a treasure trove of insights that inform business strategies and help maintain a competitive edge in an ever-evolving marketplace.
Machine Learning in Predictive Analytics
To grasp the full significance of predictive analytics today, one must understand the intersection of machine learning and how it enhances decision-making processes across various sectors. Machine learning serves as the engine driving predictive models, allowing algorithms to identify patterns within vast datasets that would otherwise remain unnoticed. This ability to process large amounts of information quickly and efficiently is not merely beneficial; it’s transformative, making it possible to predict outcomes with higher accuracy and precision than traditional statistical methods.
In an age rife with data, machine learning shines as a beacon, illuminating pathways toward smarter strategies. Organizations now leverage these techniques not just for analytical insights, but also to underpin automated decision-making systems that react in real-time—a crucial advantage in industries where every second counts.
Understanding Machine Learning Techniques
When exploring the myriad of machine learning techniques available, it’s crucial to consider a few fundamental algorithms that form the backbone of predictive analytics:
- Regression Analysis: This involves predicting numeric outcomes based on historical data. For instance, in finance, regression techniques can help forecast stock prices by analyzing past trends and associated market variables.
- Classification Algorithms: Such as decision trees or support vector machines, these methods categorize data into distinct classes. An application could be in healthcare, where patient data is classified to predict disease or health outcomes.
- Clustering Techniques: Aimed at grouping similar data points together. In marketing, these can help organizations understand distinct customer segments for targeted advertising campaigns.
While understanding these techniques is key, what’s vital is recognizing their applications and the situational nuances that determine which one is most effective. As one navigates the options, balancing complexity with interpretability becomes paramount in making informed decisions.
Applications Across Industries
The versatility of machine learning in predictive analytics extends to multiple industries, providing tailored solutions. Here’s a snapshot:
- Finance: Beyond traditional risk assessments, machine learning predicts market trends, enhances fraud detection capabilities, and optimizes trading strategies—allowing investors to benefit from nuanced market signals.
- Healthcare: From predicting patient admissions to diagnosing diseases via symptom recognition, machine learning plays a pivotal role in optimizing patient outcomes and resource management.
- Retail: By examining purchasing patterns, retailers can anticipate inventory needs and tailor marketing strategies, ensuring customers receive personalized experiences that drive loyalty.
- Manufacturing: Predictive maintenance powered by machine learning data analysis helps reduce equipment failures, boosting efficiency and saving costs.
What’s remarkable is how these applications are not standalone systems but rather interlinked. An advancement in one field often translates to breakthroughs in another, creating a ripple effect across the economy.
"The rise of machine learning is pushing the boundaries of what is knowable, allowing organizations to make proactive rather than reactive decisions."
In summary, machine learning has positioned itself at the heart of predictive analytics, refining the methodologies employed and extending their reach across diverse industries. As these technologies evolve, the implications for investors and decision-makers remain profound, underscoring the necessity of staying informed and agile in a rapidly changing landscape.
Big Data and Predictive Analytics
As we dive into the intersection of big data and predictive analytics, it becomes clear that this relationship has transformed how businesses operate. Big data refers to the massive volumes of data generated every second from various sources, like social media, website interactions, and sensors, among others. Incorporating predictive analytics enables organizations to derive useful insights from these data troves. Ones can sense patterns and trends by analyzing historical and real-time data, allowing for better forecasting, decision-making, and strategic planning.
The importance of utilizing big data in predictive analytics can’t be overstated. It is like fishing with a net rather than a line; the broader the catchment area, the more diverse the insights. Companies that only scrape the surface of their data often miss out on hidden treasures that could lead to significant improvements or innovations. By integrating big data with predictive analytics, firms can discover an unprecedented depth of actionable intelligence.
Integration of Big Data
Integrating big data into predictive analytics is akin to blending various ingredients to create a complex dish. Every data source adds flavor and texture to the final outcome. To successfully integrate big data, organizations must follow these key steps:
- Data Collection: This involves aggregating data from various sources such as IoT devices, customer interactions, and operational processes.
- Data Cleaning: Raw data can be messy. Cleaning it is necessary to ensure accuracy in analysis.
- Data Storage: Decisions around whether to use cloud storage or on-site servers must align with the company’s needs and capabilities.
- Data Processing: Utilizing tools like Apache Hadoop or Spark can facilitate the processing of colossal datasets efficiently.
- Data Analysis: Here, predictive models can be deployed to derive insights. Machine learning algorithms can analyze trends with unsurpassed precision.
Ultimately, the ability to merge various types of data is what enables businesses to predict future trends. For instance, retailers can analyze purchasing patterns alongside customer sentiment on social media to tailor their marketing efforts.
Challenges and Opportunities
While the opportunity pool is vast, the challenges posed by big data integration into predictive analytics are considerable. Here are some challenges:
- Data Privacy Concerns: With regulations like GDPR, companies must tread carefully when handling personal data. Violating these regulations can lead to hefty fines and reputational damage.
- Quality Over Quantity: Not all data is good data. Sifting through noise to find signal takes time and sophisticated tools.
- Skill Gap: There’s often a shortage of professionals skilled in both big data technologies and predictive analytics methodologies.
Yet, despite these challenges, the opportunities present a persuasive case for adaptation. Organizations can tap into:
- Enhanced Decision Making: Data-driven decisions tend to be more sound and less prone to bias.
- Personalized Customer Experiences: Knowing customer behavior allows companies to tailor experiences, leading to improved satisfaction and loyalty.
- Competitive Advantage: By leveraging predictive analytics to understand market trends, businesses can position themselves ahead of the curve, capitalizing on emerging opportunities.
"The integration of big data into predictive analytics transforms how decisions are made, shifting from instinctual choices to informed strategies."
In summary, the fusion of big data and predictive analytics is not merely a trend; it is vital for contemporary businesses aiming for success. The synergy between these two fields promises not just to enhance operational efficiency but also to foster innovation across various sectors.
Risk Analytics
Understanding risk analytics is fundamentally essential in the modern business landscape. In an era where data informs nearly every decision, having a strong grasp on risk metrics can give entities a competitive edge. Risk analytics not only identifies potential hazards but also quantifies their impact, allowing businesses to navigate uncertain waters effectively. Whether you're an investor looking to diversify portfolios or a financial advisor optimizing strategies for clients, comprehending risk analytics helps to predict and prepare for the unavoidable uncertainties that accompany financial undertakings.
Defining Risk Analytics
Risk analytics is the practice of using data analysis techniques to assess and manage risks associated with investments and operations. It involves gathering historical data, applying statistical methods, and utilizing predictive modeling to evaluate potential future outcomes. Essentially, it helps organizations anticipate risks before they materialize, which can mean the difference between profit and loss.
In this context, the following elements are central to understanding risk analytics:
- Data Collection: It starts with gathering relevant data—everything from market trends to economic indicators.
- Modeling Techniques: Various methodologies, like Monte Carlo simulations or regression analysis, are employed to simulate various scenarios and their consequences.
- Principle of Risk Assessment: This aspect revolves around understanding exposure and vulnerability to potential threats, assisting in the prioritization of risks based on their likelihood and potential impact.
Quantifying Risks
Quantifying risks refers to the process of determining the likely impact of various risks on an organization. This requires not only the identification of risks but also the measurement of their potential impact in numerical terms. Here are crucial benefits and considerations when dealing with risk quantification:
- Risk Measurement Framework: Establishing a robust framework is essential. This includes defining key risk indicators (KRIs) that are relevant to your organizational specifics. For effective measurement, adopting a consistent methodology is vital to compare risks appropriately.
- Value at Risk (VaR): VaR is one of the most used metrics, providing an estimation of potential losses in a portfolio. It's a straightforward, quantitative measure that can guide decision-making under conditions of uncertainty.
- Integrating Stress Testing: Implementing stress testing scenarios empowers managers to understand how extreme conditions would affect their operations. Pushing models to the limits can uncover vulnerabilities that routine analyses might miss.
"Risk analytics transforms the often murky waters of investment and operational strategy into comprehensible metrics that can be navigated effectively."
While quantifying risks may seem straightforward, it comes with its challenges. Data availability and quality can create roadblocks; often, the past may not be a good predictor of future risks due to market volatility or unexpected global events. However, even with these challenges, businesses that embrace and refine risk analytics find themselves far better equipped to thrive amidst uncertainty.
Forecasting Techniques
In the world of predictive analytics, forecasting techniques play a pivotal rôle in shaping strategies for businesses and investors. These techniques are not simply tools; they are windows through which one can glimpse the future. By analyzing past data and identifying patterns, companies can make educated predictions about the direction of their market, the behavior of consumers, and potential financial outcomes. Moreover, embracing forecasting allows stakeholders to navigate risk more effectively and seize opportunities before they pass.
Key elements of forecasting techniques include:
- Data Reliability: Quality data is a cornerstone of effective forecasting. An insight based on shaky data is likely to lead to poor decisions. Thus, ensuring that data collection methods are robust and reliable can't be overstated.
- Methodological Rigor: The techniques implemented must be suited to the specific context. For instance, a retail environment may demand different approaches than a manufacturing setting.
- Continuous Improvement: Forecasting is not a one-off task. Ongoing refinement based on new information and changing conditions can enhance the accuracy and applicability of forecasts.
Looking to the benefits of forecasting techniques, one can identify several notable aspects:
- Enhanced Decision-Making: By predicting future trends, businesses can tailor their strategies accordingly and make informed decisions rather than rely on gut feelings.
- Resource Allocation: Effective forecasting allows organizations to allocate resources more efficiently, minimizing waste and maximizing productivity.
- Competitive Advantage: Companies that anticipate market changes can position themselves advantageously against competitors who may be slower to adapt.
However, certain considerations must also be kept in mind:
- Complexity of Models: More sophisticated forecasting models can lead to overfitting, where a model becomes too tailored to historical data and fails to perform on unseen data.
- Uncertainty and Variability: External factors such as economic shifts, technological advancements, or sudden market changes can impact the reliability of forecasts.
Quantitative Forecasting Approaches
Quantitative forecasting approaches rely heavily on numerical data and analytical methods. These techniques are grounded in statistical models that take historical data and apply mathematical computations to uncover patterns and trends.
A few methods typical of quantitative approaches include:
- Time Series Analysis: Involves analyzing sequences of data points over time to predict future values. This could apply, say, to monthly sales figures, suggesting trends and seasonal fluctuations.
- Regression Analysis: It examines the relationship between variables, determining how changes in one or more independent variables impact a dependent variable. For instance, how price changes might affect consumer demand.
- Moving Averages: This smoothing technique reveals trends by averaging data points over a specific time frame. It’s particularly useful in identifying cyclical patterns.
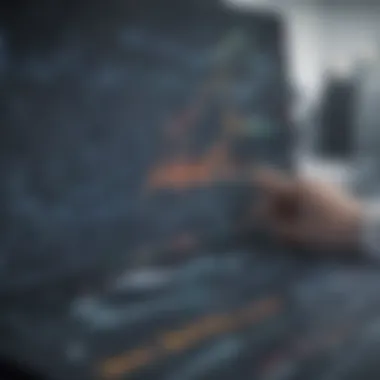
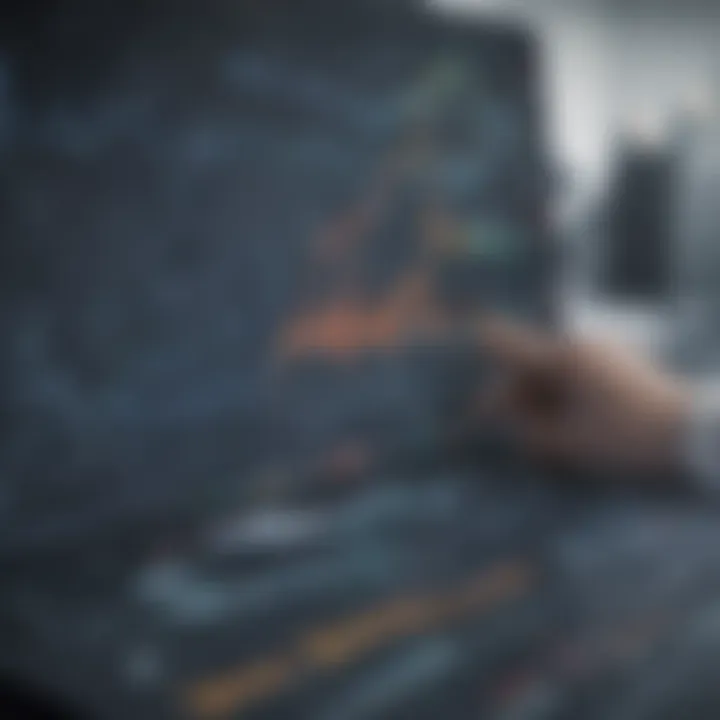
Pros of quantitative approaches are straightforward, as they provide measurable and straightforward results. However, they have drawbacks—namely, their dependency on the quality of the historical data. If past data is flawed or misleading, predictions will likely go awry.
Qualitative Forecasting Insights
While quantitative methods often get the spotlight, qualitative forecasting holds its own significant merits. Qualitative approaches rely on subjective judgment and expert opinions rather than just numerical data. They are particularly advantageous in situations where historical data is sparse or non-existent, such as predicting the success of a new product launch.
Methods used in qualitative forecasting are:
- Delphi Method: Engaging a panel of experts in multiple rounds of questioning to converge on a consensus forecast. Each round allows for revision based on feedback, refining the predictions.
- Market Research Surveys: Gathering insights directly from consumers can yield valuable qualitative data. This presents opportunities to gauge potential consumer reaction to new initiatives.
- Focus Groups: By discussing with a group of target audience members, businesses can capture nuanced insights that may not emerge in traditional data analysis.
Qualitative methods often incorporate rich details. However, they do rely heavily on the subjective experience and biases of those involved. Thus, melding both quantitative and qualitative techniques can often provide the most dependable forecasting outcomes, as each compensates for the weaknesses of the other.
"Just as a compass guides a traveler, effective forecasting techniques give businesses the foresight needed to navigate the future landscape of their respective industries."
In essence, balancing both approaches opens a wealth of potential in predictive analytics.
Implementation of Predictive Models
The implementation of predictive models stands at the very core of harnessing data's potential for decision-making. This phase transforms theoretical concepts into actionable insights. It's crucial because it defines how well the models will perform in real-world situations. By translating complex algorithms into deployable strategies, organizations can seize opportunities, anticipate challenges, and make informed decisions. Here, we delve into the specific elements involved, the inherent benefits, and crucial considerations surrounding this implementation process.
When we discuss implementation, some might think it’s just a technical burden. But in reality, it’s a strategic cornerstone. To put it simply: without proper implementation, predictive models are just smart ideas in a textbook. Firms that prioritize correct implementation reap numerous benefits, including improved accuracy, cost savings, and enhanced competitiveness. However, rushing through the steps can lead to complications down the road.
Steps in Model Implementation
To effectively implement predictive models, organizations should follow a systematic approach. Here’s a breakdown of critical steps that guide this process:
- Define Objectives: Establish clear business goals. What does the organization hope to achieve with the predictive analysis? Having specific targets in line will ensure the model's direction remains focused.
- Data Collection & Preparation: Gathering the right data is fundamental. This stage isn't just about quantity but the quality and relevance of the data collected. Data wrangling may be necessary to ensure consistency.
- Model Selection: Choose appropriate modeling techniques. Depending on the analysis' scope, different models like regression, decision trees, or neural networks could be more suitable.
- Training the Model: Once a model is selected, it needs to be trained using historical data. This is where the model learns patterns from past experiences.
- Testing and Validation: Rigorously evaluate the model’s performance using a separate dataset. Analyzing results against known outcomes helps identify potential biases and errors.
- Deployment: After validation, it’s time to go live. Implement the model within the business processes, ensuring integration with existing systems.
- Feedback Loop: The work doesn't end post-deployment. Establish channels for continuous feedback to refine and optimize the model over time.
Following this structured approach can help organizations avoid pitfalls and misunderstandings that could arise in the modeling process. A well-implemented model can lead to actionable insights that significantly impact strategy and decision-making.
Monitoring and Evaluation
Once the model is in place, it doesn't just sit there. Ongoing monitoring and evaluation are paramount to ensure the predictive analytics remain relevant and effective. Here’s why it matters:
- Adapt to Change: The business landscape is dynamic. Regular assessments help models adapt to new patterns or variables frequently emerging in the data.
- Performance Metrics: Establish specific metrics to evaluate the model's performance. Metrics, such as accuracy, precision, and recall, allow organizations to quantify the success of their predictive efforts.
- Adjustments and Enhancements: Continuous feedback supports adjustments that can enhance model performance. Sometimes, it necessitates retraining the model with fresh data or modifying existing parameters.
"A predictive model is only as good as its last evaluation."
Incorporating a systematic monitoring and evaluation framework is a must for organizations looking to maintain a sharp competitive edge.
As organizations increasingly rely on predictive models, understanding these implementation processes and the need for constant evaluation can empower decision-makers. They can harness the true value of data analytics, leading to effective strategies and informed choices.
Ethical Considerations
The realm of predictive analytics brings with it not just the promise of insights but also a plethora of ethical considerations that cannot be ignored. As these advanced analytics methods become integral to decision-making processes across industries, understanding the ethical implications is paramount. Ignoring these considerations could result in significant risks to privacy, fairness, and accountability. Stakeholders—be it investors, financial advisors, or entrepreneurs—must consider how their data-driven strategies align with ethical standards. This section examines the critical aspects surrounding ethical considerations in predictive analytics, focusing on specific elements like data privacy and bias.
Data Privacy Issues
Data privacy stands as a cornerstone in the ethical framework of predictive analytics. With organizations amassing vast amounts of data, they find themselves in an intricate dance of balancing the benefits of analytics against the rights of individuals. Many users are often unaware of how their data is collected, stored, and utilized. This disconnect can sow distrust and even lead to regulatory scrutiny.
The importance of protecting personal information cannot be overstated. Legislation such as the General Data Protection Regulation (GDPR) has thrown a spotlight on data privacy, requiring organizations to adopt transparent data practices. For an investor, understanding these regulations is vital; violations can lead to hefty fines and damage to reputation.
- Transparency: Companies should openly communicate about data collection practices. When people know how their info is used, it fosters trust.
- Consent: Obtaining user consent is no mere formality. It’s a crucial step in ethical data handling. Users should have control over what data they share.
- Anonymization: Where feasible, anonymizing data can mitigate privacy risks, allowing organizations to utilize insights without compromising individual identities.
Thus, navigating the terrain of data privacy not only aligns with ethical practices but also enhances the long-term sustainability of an organization’s analytics endeavors.
Bias in Predictive Analytics
Bias in predictive analytics is a thorny issue. As the saying goes, "Garbage in, garbage out." If the data used to train predictive models contains biases, the resulting analytics will exhibit those same problematic trends. For instance, consider a hiring algorithm trained on historical data where certain demographic groups were underrepresented. If unchecked, such bias can perpetuate discrimination, affecting opportunities for numerous qualified candidates.
The repercussions of algorithmic bias are far-reaching, influencing everything from lending decisions to healthcare diagnostics. Investors and financial advisors should recognize that a seemingly fine-tuned model can harbor biases that lead to flawed business decisions. Addressing this concern involves several steps:
- Diverse Data Sets: Organizations should aim to utilize varied data, reflecting the population they serve. This helps ensure that the insights generated are more representative.
- Regular Audits: Implement regular bias audits of predictive models. These audits can unveil hidden biases enabling firms to proactively correct inequities.
- Human Oversight: Relying solely on algorithms can be misleading. Continuous human oversight ensures that unethical bias can be caught and addressed before it impacts decisions.
"Inaction in the face of biased analytics is not just a missed opportunity; it's a step backward for society."
Future Trends in Predictive Analytics
In an era where data is increasingly seen as the new oil, the future trends in predictive analytics hold promise for investors and businesses alike. These trends are not just evolutionary but also revolutionary, reshaping how industries operate and make data-driven decisions. Understanding these advancements is vital for anyone who aims to stay competitive in a fast-paced financial environment. By tapping into the future of predictive analytics, organizations can expect to enhance decision-making, optimize operations, and ultimately improve their bottom line.
Technological Innovations
The landscape of predictive analytics is being propelled forward by several technological innovations that enhance data processing capabilities and analytical proficiency.
- Artificial Intelligence and Machine Learning: These technologies are at the forefront, enabling systems to learn from historical data and make predictions with minimal human intervention. For instance, companies like Google use machine learning to predict user behavior, tailoring ads based on anticipated interests, thereby increasing both engagement and revenue.
- Cloud Computing: The shift to cloud-based solutions allows for greater scalability and efficiency in storing and processing vast amounts of data. Services like Amazon Web Services provide on-demand resources that make running complex predictive models much easier. With cloud computing, even small enterprises can utilize powerful analytics tools without hefty upfront investments.
- Real-Time Analytics: The demand for quicker insights is escalating. Organizations are investing in real-time analytics solutions that allow decision-makers to act promptly based on the latest data. For example, in the finance sector, firms use real-time analytics to monitor stock trends and make immediate trades based on predictive insights, maximizing their portfolio performance.
"Predictive analytics is not just about knowing the future; it's about preparing for it."
Embracing these technological advancements shapes a dynamic framework where predictive analytics becomes accessible to a broader audience, fostering an environment ripe for innovation.
Emerging Industries
New industries are beginning to adopt predictive analytics, and some unexpected sectors are harnessing its power to gain a competitive edge. Understanding these trends can reveal unique investment opportunities and insights.
- Healthcare Sector: Predictive analytics plays a pivotal role in patient care and medical research. Hospitals are using data-driven approaches to predict patient admissions and optimize staff allocation. Organizations focused on preventive care leverage analytics to identify at-risk populations, thus improving overall public health outcomes.
- Agriculture: Farmers are turning to predictive analytics to anticipate weather changes and optimize crop yields. Technologies such as IoT devices collect real-time data from fields, allowing farmers to make informed decisions about irrigation and fertilization. This trend promotes sustainability while improving profitability.
- Retail and E-commerce: The retail industry uses predictive analytics to enhance customer experiences through personalized services. By analyzing purchasing behaviors, retailers can forecast inventory needs and tailor promotions to specific demographic groups, thus maximizing sales potential.
As these emerging industries embrace predictive analytics, they open doors for investors to explore new avenues. Staying abreast of these developments could prove advantageous, as the application of predictive insights expands far beyond traditional sectors.
End
As we wrap up this comprehensive exploration of predictive analytics, it becomes clear just how pivotal these methods are in today's data-centric landscape. Understanding the various types of predictive analytics not only broadens one’s knowledge base but also serves as a practical toolkit for making more educated decisions, particularly in financial contexts. Predictive analytics offers a lens through which data transforms into actionable insight, guiding both investors and analysts alike through the chaotic waters of economic forecasting.
Summary of Important Concepts
Throughout this article, we have dissected several critical aspects of predictive analytics:
- Types of Predictive Analytics: Each type, from descriptive to prescriptive, exhibits distinct characteristics and applications.
- Techniques and Tools: The methodologies employed range from statistical analysis to machine learning frameworks. This blending of techniques enhances predictive power and accuracy.
- Importance of Data Quality: The success of predictive models largely hinges on the quality of input data. Garbage in, garbage out, as the saying goes. Ensuring the integrity and accuracy of data can make or break analytical efforts.
- Ethical Considerations: Navigating the ethical landscape of predictive analytics is not just advisable but necessary, especially regarding data privacy and bias correction.
- Future Trends: With rapid technological advancements, the evolution of predictive analytics is a constant. Awareness of upcoming trends can position users ahead of the curve, making them more competitive.
These elements combined create a foundation upon which effective decision-making can flourish. Educators, businesses, and financial entities must embrace these insights to drive more predictable outcomes.
Implications for Investors
In the world of investment, having a finger on the pulse of predictive analytics is not merely advantageous; it’s indispensable. Investors who grasp the nuances of these analytics stand to gain a competitive edge. Here’s how:
- Informed Decision-Making: Investors can leverage predictive insights to shape their strategies. Utilizing data analysis to forecast potential market movements helps in optimizing portfolio choices.
- Risk Management: Understanding risk analytics allows for the identification and quantification of potential threats. Anticipating risks leads to strategic positioning in volatile markets.
- Performance Evaluation: By measuring outcomes against predictive models, investors can refine their strategies continually. Learning from predictive outcomes cultivates an adaptive investment approach.
- Emerging Opportunities: Stay alert to technological advancements that can affect market conditions. Those who can identify and adapt to these changes are likely to outperform their more passive counterparts.
Ultimately, the significance of predictive analytics in investing is more than just about trends or numbers. It's about embedding scientific rigor into the art of investing to predict, adapt, and excel. As the landscape continues to evolve, so too must the tools and approaches of those engaged in it.